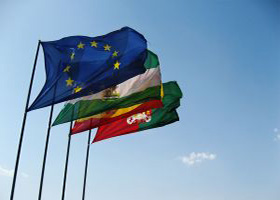
(Source: SSRN) Understanding and quantifying the evolution of security co-movements is critical for asset pricing and portfolio allocation. In international finance, the traditional case for international diversification benefits has relied largely on the existence of low cross-country correlations. Initially, this literature studied developed markets, but over the last two decades much of the focus has shifted to the diversification benefits offered by emerging markets.
In this literature, two critical questions, with important implications for asset allocation and international diversification, are of special interest for academics and practitioners alike. First, how have cross-country correlations changed through time? Using a multivariate factor model, King, Sentana, and Wadhwani (1994) did not find evidence of increasing cross-country correlations for 16 developed markets (DMs) during the period 1970-1988, except around the market crash of 1987. Longin and Solnik (1995) estimate bivariate GARCH models and Önd increas- ing correlations among seven major DMs over the period 1960-1990.
Using a GARCH-in-mean methodology, Carrieri, Errunza, and Hogan (2007) do not find a common pattern in the correlation trend for eight emerging markets (EMs) during 1977-2000. Eiling and Gerard (2007), using an alternative estimation approach, find an upward time trend in co-movements between 24 developed markets but not between 26 emerging markets over the period 1973-2005. Bekaert, Hodrick, and Zhang (2009) investigate international stock return co-movements for 23 DMs during 1980-2005. Based on a factor model, they find evidence for an upward trend in return correlations only among the subsample of European stock markets, but not for North American and East Asian markets. In summary, the evidence on the evolution of cross-country correlations over time is mixed. Most papers use factor models or a particular multivariate volatility model, and the choice of model itself may lead to different empirical findings.
The second question is if correlation is a satisfactory measure of dependence in international markets, or do we alternatively need to consider different dependence measures, notably those that focus on the dependence between the tails of the distributions? This question is narrowly related to the analysis of changes in correlation as a function of business cycle conditions or stock market performance. Following the seminal paper by Longin and Solnik (2001) and the corroborating evidence of Ang and Bekaert (2002), the hypothesis that cross-market correlations rise in periods of high volatility has been supplanted by the notion that correlations increase in bear markets, but not in bull markets. Longin and Solnik (2001) use extreme value theory in bivariate models for the U.S. with either the U.K., France, Germany, and Japan during 1959-1996. Ang and Bekaert (2002) develop a regime switching dynamic asset allocation model, and estimate it for the U.S., U.K., and German system over the period 1970-1997. Both papers estimate return exceedances at predetermined threshold values, i.e. they define the tail observations ex ante, and then compute unconditional correlations for the tail for a small sample of developed markets.
This paper substantially contributes to our understanding of both these important questions. Regarding the patterns and trends in correlations over time, much of the existing work suffers from limitations endemic to the model and/or methodology used. Many studies use factor models, and the correlation estimates are obtained as a by-product of the analysis of the factor model. Estimation results are therefore conditional on the validity of the factor model, which may be problematic given the limited explanatory power of these models. Moreover, estimation of multivariate GARCH models has traditionally been technically challenging when using large numbers of markets, due to the large number of parameters that need to be estimated, which may lead to convergence problems in optimization. In this paper, we characterize time-varying correlations using weekly returns during the 1973-2009 period for a large number of countries (either thirteen or seventeen EMs, sixteen DMs, as well as combinations of the EM and DM samples), without relying on a factor model. We implement models that overcome the dimensionality problems, and that are easier to estimate. To do so, we rely on the variance targeting idea in Engle and Mezrich (1996). Since in large-scale estimation, parameters that drive the covariance dynamics are estimated with bias, we implement the numerically more e¢ cient composite likelihood procedure proposed by Engle, Shephard and Sheppard (2008). We use the more sophisticated dynamic conditional correlation (DCC) model of Engle (2002) and Tse and Tsui (2002), as well as the dynamic equicorrelation (DECO) model of Engle and Kelly (2008) that can be estimated on large sets of assets using conventional maximum likelihood estimation. We also contrast our findings with those obtained using the more traditional scalar BEKK model. We thus demonstrate that it is possible to estimate correlation patterns in international markets using large numbers of countries and extensive time series, without relying on a factor model that may bias inference. Our implementation is relatively straightforward and computationally fast, which allows us to report results using several estimation approaches, while assessing the robustness of our Öndings.
Download the full paper.