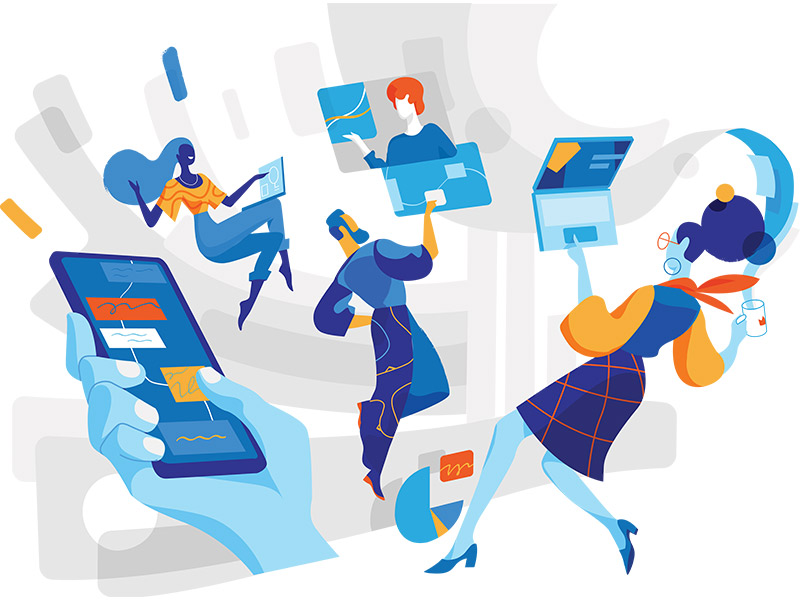
In 2016, the Toronto Transit Commission sued its insurer for allegedly not having appropriate benefits fraud controls in place to detect unusual trends or patterns.
The lawsuit was settled three years later. In the meantime, 10 people, including nine TTC employees, were convicted in relation to the scheme and more than 250 TTC staff resigned or retired to avoid dismissal or were dismissed outright, while an additional 14 staff were disciplined. As a commander with Waterloo, Ont.’s police force, Gary Askin (who wasn’t involved in the TTC case) saw similar cases firsthand, involving employees who participated without any awareness of the severity of the act they were committing or the potential consequences.
“[Plan members] don’t understand [benefits fraud] because nobody’s ever spoken to them about it,” says Askin, assistant vice-president of fraud and risk management at Sun Life Financial Inc. “Canadian companies know what to do when somebody steals a laptop from the workplace, but when somebody commits benefits fraud, some of them look at it as something different. We’re saying, it’s really not, it’s stealing from the workplace.”
Read: Toronto Transit Commission settles benefits fraud lawsuit
TTC case by the numbers
• $5M — The amount in damages the TTC was seeking from its insurer for negligence, negligent misrepresentation and breach of contract for all losses incurred
• 600 — The number of TTC staff who were under investigation for their involvement
• 10 — The number of people, including 9 TTC employees, who were convicted for the scheme
In the years since the TTC case made headlines, the fight against benefits fraud is evolving, thanks to new partnership efforts that are unifying the resources of various insurers and deploying the latest technology tools.
New tools
Indeed, insurers are increasingly relying on joint claims investigation models and the use of artificial intelligence, which is assisting in the discovery of concerning patterns across large pools of data, says Joanne Bradley, vice-president of anti-fraud at the Canadian Life and Health Insurance Association.
In 2021, the CLHIA introduced a provider alert registry, which allows insurance companies to review the benefits frauds investigations conducted by their industry peers. In 2022, it launched a data pooling system that’s supported by AI and analyzes large sets of claims data from all of the insurers aligned with the CLHIA. The AI can determine patterns of potential fraud, says Bradley, enhancing the effectiveness of these investigations. And the CLHIA’s third tool, introduced in 2023, is a joint fraud investigation program, which enables organizations to work together through a framework to share and conduct joint investigations on suspected benefits fraud cases impacting more than one insurer.
The CLHIA has seen a growth in the number of insurers and employers using the tools. “[We] certainly envision continued use, expansion of use and expansion of each one of those programs over time,” says Bradley.
The CLHIA’s data pooling system is supported by the technological capabilities of provider Shift Technology, a firm specializing in AI tools. The tool is based on generative AI, which is capable of learning patterns and creating new content like text or images.
Read: CLHIA launching initiative to pool data, use AI to detect benefits fraud
The most effective way to describe AI’s role in preventing benefits fraud is that it serves as a tool for claims handlers or investigators to deploy and comb through massive pools of data that would otherwise take them significantly longer, says Mark Starinsky, Shift’s senior product manager of payment integrity.
But, he adds, it isn’t just about reading the information alone. “AI isn’t just able to handle vast amounts of data very, very quickly; [it’s] also able to pattern out things.”
The ability to discern these patterns is one of the most critical elements in identifying benefits fraud and that’s where generative AI comes into play, he says, noting Shift relies on its AI tool to identify connections between the subjects its reviewing to try to discover suspicious behaviour and activity. It then runs algorithms against these connections — for example, between doctors, patients, a facility, a claim or a policy — and tries to identify anything nefarious. “Maybe there’s an inordinate relationship between a doctor and a certain laboratory, which may infer that there may be a kickback scheme going on between those two,” says Starinsky.
Technology tools
• Artificial intelligence and machine learning — These tools help insurers analyze patterns in large datasets to detect anomalies indicative of fraudulent activities.
• Robotic process automation — This tool automates routine tasks and reduces human error, which can be exploited by those behind fraudulent schemes.
Shift’s large language models can comb through human text and be trained to detect suspicious inconsistencies that could identify a fraudulent scheme. “If a health plan has medical records, we can analyze those documents and point to where there are differences or anomalies between what the records say versus what was documented in a structured text on the claim. We do this with plan policies where we can extract information out of a policy and actually make that coding logic.”
AI and insurers
Traditionally, insurers have relied on predetermined scenarios and business rules to identify suspicious plan member activity, says Askin, which resulted in organizations lagging behind the latest trends.
In the past, once a trend was detected, it would take too long for changes to be implemented, he adds, which led to extended fraud claims going unnoticed.
Read: AI tools helping insurers manage plan costs by weeding out instances of benefits fraud
However, AI and machine learning tools are shaking things up. Sun Life has developed an AI and machine learning service that aims to be flexible and continuously adapt to new patterns based on feedback from human investigators. The tool, says Askin, provides investigators with a holistic profile of the benefits claimant. “We’ve been getting some great success with [the AI learning tool] because . . . our investigators are combing through hundreds of millions of claims, [which is] difficult to do without this type of AI and machine learning.”
The biggest advantage of AI and machine learning tools is how much data they can review and the speediness of doing so, says Chad White, director of corporate security at Medavie Blue Cross. “You can [ask] a robot to look for specific scenarios that might be indicative [of fraudulent activity] and so it just crawls through the dataset, looking for those alerts and then it will highlight that for an audit investigator. Then they can pick up that case and see whether or not it’s a false positive or whether or not there’s really something here that we need to investigate further.”
The speed of AI tools is critical for insurers, he notes, since most pay their claims in 24 to 48 hours, leaving a small window of time to identify any issues. “Historically, when we were just doing samples, you might have missed something or you didn’t see something because you either picked that sample or you didn’t. But now, these modern tools allow us to do this much faster than a human could.”
The first step
Key takeaways
• In recent years, the CLHIA has introduced new programs and tools that are prioritizing industry collaboration and relying on emerging technologies such as AI.
• AI, in particular, is opening the doors to a new landscape of fraud detection since it cancomb through significant pools of data quickly and efficiently.
• Despite all of the technological advancements, benefits fraud prevention ultimately starts with awareness from all parties involved.
No matter how advanced the technology, benefits fraud prevention has to start with an awareness from plan sponsors and members, says Bradley, noting the CLHIA offers digital training options around effective reporting and understanding fraud.
Indeed, White says it falls on insurers and plan sponsors to create an environment of information that can meaningfully affect change. “How can we work together to make sure that everybody understands that committing the fraud isn’t cheating a billion-dollar company, it’s cheating your own organization and the sustainability of your plan.”
Read: CLHIA working with insurers on suspected benefits fraud investigations
In 2019, the Ontario government launched the Serious Fraud Office, which investigates and prosecutes increasingly complex white-collar crime. Sun Life meets with this government agency every year to share information about benefits fraud prevention tactics. Similarly, the insurer shares expertise in a subcommittee of the Ontario Association of Chiefs of Police that includes private, public and police groups.
“The best weapon we have against people committing fraud is not technology, it’s an informed public if they’re aware that individuals will lose benefits,” says Askin.
The Canadian market’s approach to fraud has improved significantly in recent years, says Starinsky, crediting the CLHIA’s leadership, as well as the collaboration effort between insurers. However, he wants to push things even further and has had conversations with Shift’s Canadian partners to deploy more detection test scenarios.
In terms of the adoption of AI, White acknowledges the industry is in its infancy. Looking ahead, he’s excited for future capabilities as more and more data is collected. With these new solutions, his team is converging their fraud analytics, data science and fraud investigation efforts.
“The more data that [it] consumes over the next few years, the smarter and faster these tools and more helpful those tools will become because they’re more informed. [The AI will] have much more data to figure out patterns and anomalies and be able to tell us a lot quicker when something has gone sideways.”
Bryan McGovern is an associate editor at Benefits Canada and the Canadian Investment Review.