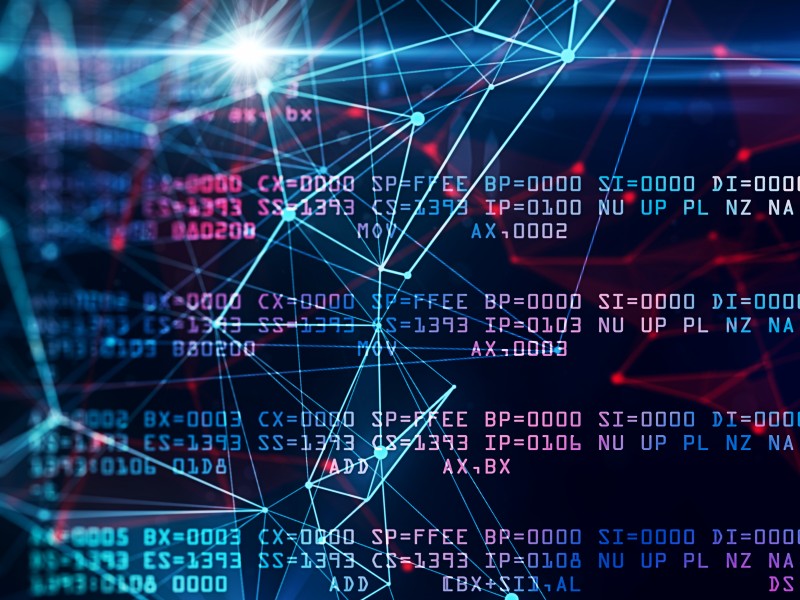
Machine learning can be a useful tool when analysizing public equity options, but users need to understand and mitigate the limitations of the technology, according to research published in the Financial Analysts Journal.
The term machine learning is a catch-all for algorithms and methods that allow machines to uncover patterns without specific programming instructions, the research noted.
“Machine learning algorithms can be useful even with noisy datasets, like stock data, if you’re very careful about how you set the problem up,” says the report’s co-author Robert Jones, chief investment officer and co-founder of System Two Advisors.
It can also can help investors solve problems that human beings are inherently less capable of solving, given our propensity for linear thinking, says Jones.
“Our brains are limited in our ability to uncover patterns,” he says. “We are pattern-seeking animals, but as the same time, we tend to think in a linear fashion, and we sometimes don’t pick up on things that algorithms might be able to capture.”
For example, the common consensus is that stocks with low price to equity ratios perform better than those with high ones. As a result, investors have a tendency to seek out lower P/E ratio equities, says Jones. “They look for low P/E stocks, that’s our human, linear brain. But a machine brain might be able to say, ‘Well, low P/E stocks do better in certain environments, in certain sectors of the economy, less volatile stocks,’ whatever it is, that can be more conditional in a non-linear sense. It depends on the nature of the stock and the market environment: sometimes low P/E stocks do well, sometimes they don’t.”
Examining how various factors like these coincide can create a deeper well of knowledge on which to base equity selection, for investors seeking to add alpha through more active management techniques, says Jones.
There are, however, limits to the tools that investors should factor into the resulting analysis, says Keywan Rasekhschaffe, senior quantitative strategist and portfolio manager at Greshem Investment Management, co-author of the report. Patterns can appear that aren’t actually helpful for predictive analytics, resulting in overfitting modelling errors, he says. When inputting over 100 different factors in a model, their relationships with each other can become quite complex, he notes.
A pitfall of using machine learning and analysis is that an algorithm can only account for the facts in the data it is given, he says. “If one factor in our factor library has much more overfitting bias than another, the algorithm doesn’t know that. And it might pick this very well-performing example factor, but it might be much more overfit. And that’s something you really don’t want.”
Human judgement is needed to properly curate what factors to include, he says, ideally including factors that have low bias when viewed alone, but also relative to other factors included.