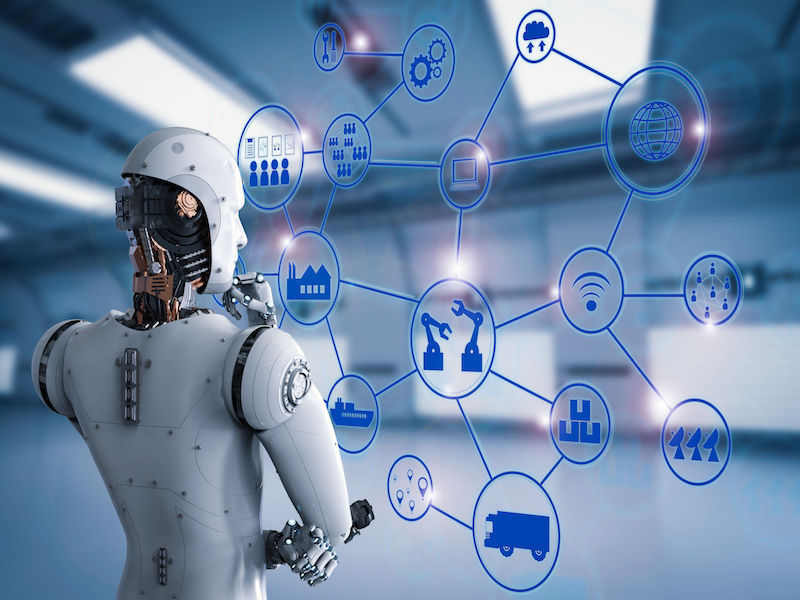
Traditionally, all finance students had to learn statistics, and, in the future, this will likely shift into finance students requiring machine learning, said John Hull, professor of finance and Maple Financial Group Chair in derivatives and risk management at the University of Toronto’s Rotman School of Management.
“It’s not that our students are actually going to become data scientists — they’re not — some of them might, but most of them won’t. But they’ve actually got to know how to talk to data scientists, how to make sure the data scientists are doing things which are useful to their organizations,” he noted, speaking at Canadian Investment Review‘s 2019 Risk Management Conference.
Machine learning is one area of artificial intelligence, which is about learning from data. “We give a computer program . . . access to volumes of data and it learns about relationships between variables and makes predictions.”
It is a natural development from statistics, as it involves huge data sets. Yet, the language in statistics and machine learning is totally different, Hull noted. “In fact, I haven’t found a single word in machine learning that has the same meaning in traditional statistics.”
Interestingly, machine learning isn’t new and it actually dates back to the 1950s. Yet, the reason there’s so much hype now is because there is a huge amount of data available and computing is cheaper than it once was.
When applying machine learning, investors need to be cautious of overfitting or underfitting data, Hull said.
So how does portfolio management fit into the discussion about machine learning? This can either be done through supervised learning or reinforcement learning, he said.
Supervised learning is making a single decision. In application, this would be looking at different features and deciding to increase exposure in a certain area, for example, Hull said.
And reinforcement learning is about making sequential decisions and interacting with the environment. “Reinforcement learning is a lot trickier. You need a lot more data for reinforcement learning and you can very quickly run into problems in terms of the number of features and that sort of thing.”
Some machine learning best practices include dividing data into training, validation and test sets, developing different models using the training set, and comparing them using the validation set and choosing the most accurate one, Hull noted.
According to Hull, some key questions investors should ask when shown the results of machine learning include: What was used as the training data set? How many different models were tried? What were the results? What was used as the validation data set? How well did the results for different models work for the validation set? What was used as the test data set, and what results did it give? And, is the model chosen stationary, or does it evolve with the market?
Despite the growing prevalence of machine learning, managers need not fret. “This isn’t going to replace you as managers, let me say this. But it may lead to tools which become increasingly useful to you as portfolio managers,” Hull said.