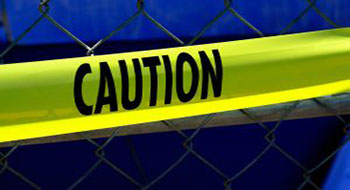
In the world of pensions and investments, we often find ourselves caught up with the “number.” Whether it’s market value, a risk metric or a prediction, the outcome focuses our attention on an exact value, often without context as to its variance. This can lead to an illusion of precision, imputing significance where the opposite is the case and adversely affecting our investment decisions.
Predictions
The most obvious example comes in the area of predictions. Analysts are constantly offering predictions for equity indexes, individual equities and commodity prices. These predictions often use models that provide exact values for the outcome. But rather than blindly relying on the value, we intuitively focus on the direction and magnitude.
For example, given a year-end prediction of 1,205 for the S&P 500, we would not deem the prediction poor if the S&P 500 closed at 1,212. We focus on the starting value of the index, the predicted direction and magnitude, and the inherent volatility of the index, then we adjust our assessment of the prediction’s accuracy to those inputs. We do this intuitively, and it is unlikely to affect our investment process.
Risk limits
The field of risk management uses complicated relationships between securities or asset classes to predict worst-case scenarios.
For example, a diversified fund of $1 million with investments in several asset classes is managed with a risk budget. This budget can be expressed as a 5% probability of losing more than a material amount, such as $100,000, in one month. The $100,000 value is itself based on prospective returns, as well as volatilities and correlations of these assets, which are usually based on historical data.
Even though we can state the probability of a loss with some precision, this accuracy is based on assumptions that are subject to inherent limitations; therefore, a good risk manager will determine the sensitivity of the model’s output to the inputs.
Furthermore, a system of acceptable risk is not based on a single number but on threshold levels with varying degrees of acceptability. In the “traffic-light” approach, a designated risk level (such as 5% risk of a $50,000 loss in our example) gives the green light to accept further risk. At $75,000, a yellow light indicates caution: it is preferable to decrease risk at this level rather than to add risk. At $100,000, the red light goes on, signalling the need for risk reduction.
By reducing reliance on a single number, the tiered risk system avoids the impression of precision and better reflects the many assumptions implicit in such predictions.
Liability cash flow projection
One final example of the dangers of overreliance on precision is the pension plan cash flow projection. These projections rely on assumptions such as mortality, salary growth and inflation, among others.
Simply assuming that the precision of the calculation infers “truth” could lead pension investors to exactly match the liability cash flows with fixed income investments and congratulate themselves on slashing the risk of the fund to zero. Clearly, this is unrealistic.
Liability cash flows are simply expected values and can fluctuate due to changes in longevity, early retirement by employees or salary increases. Moreover, a small number of high-earning pension plan members can also distort cash flows through single events that do not match the assumptions. Perhaps the high-earning retirees will enjoy robust health and long lives, resulting in higher benefit payments from the plan and revealing a mismatch between the asset and liability cash flows that would not have existed at the prior cash flow projection date. In such a case, precision in the matching of the assets and liabilities leads not only to false hopes that risk has been eliminated but also to regular adjustment of asset cash flows to maintain the cash flow match.
It is important to recognize the variability of the liability cash flows due to their underlying assumptions and to structure investments that hedge the broader risk of the liability cash flows without overreliance on the precision of the data.
In some of the above examples, such as prediction, we intuitively dismiss the precision of outcome, while in the others, we may be tempted to rely on exact figures. Either way, we can help to mitigate this reliance on precision by doing the following:
- testing outcome sensitivity to initial variables;
- modifying behaviour (and therefore expectations of outcome) by transitioning from a focus on a single number to a tiered system in the risk world; and
- focusing on broader interest rate risk rather than precise cash flow matching in the liability world.