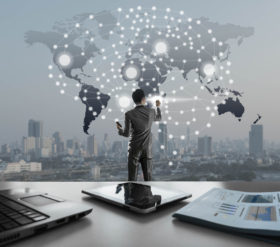
Seventeen years after Steven Spielberg’s A.I. Artificial Intelligence, the concept of AI has evolved from a figment of our imagination to a topic that is heavily discussed with its current and increased use in the financial services industry.
While much of the initial hype around AI focused on flashy robotics, many of the advances made in its applicability to businesses and end users pertain to business process improvement – moving AI from the realm of science fiction to business reality.
Algorithms are already a major play in financial market trading and there are advances being made through the use of deep learning techniques. As such, we are anticipating a new wave of managed funds, with robotics driving the analysis, security selection and trading process.
Within an environment of increasingly evolving technology and changing user expectations, the institutional investment industry is facing pressure to lower costs and improve operational efficiencies. AI and its subset, machine learning, offer institutional investors a means by which to keep pace by placing the burden on AI to drive down internal costs and improve internal operations.
Many of the industry’s more advanced institutional investors have already caught onto the value of implementing AI. They are investing in AI and machine learning to help improve investment decision making, including its support of investment research, security selection in private assets, performance attribution and portfolio construction, and talent management.
Aspects such as data ownership, bias, and accountability of AI algorithms open up wide concerns. Data, in many respects, serves as a double edged sword: while mining customer data can identify opportunities for value-add products and tailored solutions, the challenges of certain organizations to satisfactorily protect data in an environment of increasing security threats and regulations can erode the service provider-client trust sought in mining the data to begin with. Further, the ownership of data can be complicated by partnering with third parties to collect, process or store data; mergers and acquisitions can similarly muddy the waters as to how data should be valued in M&A deals, in light of its potential future value.
Data in and of itself also opens up risk in that any data set could contain the seeds of later bias; how do we then ensure machines, learning from such data sets, do not take unwanted and unanticipated actions? And, along these lines, how do we ensure transparency into what an AI program has learned and why it does what it does – and who is ultimately accountable for the results? Increasingly, AI algorithms are able to act independently, making it difficult to assign responsibility to humans for AI decisions or actions.
From the beginning, one of the primary concerns surrounding AI has been the labour market and the potential loss of jobs. In the financial industry, among others, one of the first areas in which AI implementation has been explored and implemented is customer service, including AI chatbots and virtual assistants. As a result, organizations will face challenges from growing fears of job uncertainty, while those with the ability to concentrate on offering job openings and training relating to value creating roles (roles in which AI could play an auxiliary role while not removing the need for human creativity and thought processes) may well come out ahead of their competition – both in terms of attracting new talent and long-term sustainability.
With these concerns in mind, Canada has taken a measured approach to the implementation of AI, focusing greater attention on the ethical questions behind the technological possibilities. As a global leader in ethical AI, the Financial Post reported that according to a new report on AI, almost three-quarters of Canadian firms have AI ethics committees in place; positioning them to opine on important issues such as consent, transparency, bias, and job impact alongside technology development. According to the Forbes Insight survey carried out in conjunction with SAS Institute, Intel Corp. and Accenture Applied Intelligence, Canada is also leading in ethics training, with 67 per cent having ethics training for technologists, compared to 59 per cent in the U.S. This focus in the private sector is strongly supported by the Canadian government, who have taken the stance that any algorithm used to service a citizen in Canada needs to be transparent versus standing alone as a ‘black box.’
In addition to Canada’s CIO Strategy Council, which expressed a willingness to create standardized policies to help Canadian businesses and governments, the Canadian government is working on its Pan-Canadian Artificial Intelligence Strategy with one of its goals being to develop ideas around the economic, ethical, policy and legal implications of advancements in AI. There may well be financial incentive associated with the introduction of ethics training; for example, the Forbes Insight study found that leaders who reported AI implementations to be “successful” or “highly successful” were also leading the competition in terms of ethics training for their technologists.
Investment Potential
From automating routine tasks to making recommendations based on behavior patterns, AI and machine learning offer many benefits for institutional investors. By mining vast data troves, it can help understand and predict financial behaviour and aid investment decision-making.
Online fraud and other scams are pervasive. With machine learning, it’s possible to anticipate and prevent fraud by analyzing and monitoring risk in real-time. Machine learning tools can verify identity, authorize payments, and set account limits. In addition, these tools and applications can replace high-maintenance, rules-based fraud management with self-learning algorithms to identify new patterns. They can also learn to discover exceptions, breaches of regulations, market abuse, and non-compliance.
For plan sponsors, AI and machine learning allow for the processing of vast quantities of financial data, which can then be used to monitor stock market trends. With this information it’s also possible to automate routine tasks such as rebalancing trades, monitoring risk, and ensuring compliance. And by scheduling reminders about deadlines and tax strategies, plan sponsors can encourage participants to take an active role in their retirement accounts.
Looking into the future, global AI trends allude to impacts on investment style: from fully AI-managed portfolio options potentially playing well into smart beta, to the millennial generation’s interest in ESG and community engagement in general.
Conclusion
Looking to the future, AI has the ability to transform the institutional investment industry by improving routine business processes and decision making while staying responsive to the evolving needs of end users.
While challenges relating to AI exist, both the significant investment made in this emerging technology, and the benefits seen in recent applications, indicate that AI is less a part of our collective imaginations as fixed in our future and forward looking initiatives.