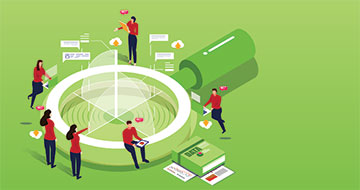
While estimating the longevity of a pension plan’s membership isn’t a new concept, developments in using big data and new tools in predictive modelling could go a long way to making predictions more accurate.
“Traditionally, most benefit plans were using standard mortality tables and not really differentiating how much longevity risk they had,” says Manuel Monteiro, a partner and leader of Mercer Canada’s financial strategy group. “So a plan covering university professors might have used the same mortality tables covering miners in Sudbury. It’s quite obvious that professors are going to live longer than miners, but many pension plans, if you look back a decade ago, might have valued their liabilities exactly the same way.”
Read: Pension stakeholders call on feds to remove barriers to longevity risk pooling
Actuaries and consulting firms are helping pension plans paint a clearer picture of their risk as these organizations better understand mortality drivers and collect data to illustrate them, he says, referring to postal codes, job descriptions and locations, education levels and pension amounts. “And basically using big data techniques to figure out what are the key predicative drivers of longevity.”
Knowledge is power
Data and analytics are major buzz words lately, says Brent Simmons, senior managing director and head of defined benefit solutions at Sun Life Financial, noting it’s been revelatory for the industry to see how certain data can be harnessed for this purpose.
“We took a really broad-based approach. . . . We broke it down into white collar, blue collar and grey collar — that middle of the road, average longevity,” he says. “You might wake up one day and think, ‘I’d really like to know whether these folks were more blue collar or more white collar.’ That was something we never recorded, so we have to go back and look at the name of the plan and some of the other characteristics that we had, and try to deduce whether they were blue or white or grey.”
A person’s affluence is a major indicator of how long they may live, says Simmons. However, he notes there are other ways to determine a reasonable assumption without knowing an exact income level. “We started to keep records of different characteristics, and within a few years we started to use postal codes as an indicator. It’s a proxy for socio-economic status, with the idea being the better off people are, usually the more educated they are about their health, the more they’re going to go to doctors or exercise or eat healthier, and therefore the longer they’re going to live.”
Read: Longer living Canadians need option of standalone longevity insurance: report
That data was combined with information regarding the pension membership’s line of work, which can be varied within a single plan, and then added to a predictive model, says Simmons. “By that time we had enough data that it made sense to throw it all into a model and crunch it, and we came out with a single model that was able to take all of these different factors and all of the data we’ve been collecting and come up with a longevity assumption.”
Canadian pension plans are already gathering the necessary data. “We participate, in terms of providing our membership data and our history, along with other additional information,” says Phil Rivard, vice-president of pension policies and funding at Alberta’s Local Authorities Pension Plan. “Not personal information, but things like postal codes for all of our members. And the third party uses that to align with a lot of other public sector pension plans in Canada to then do that predictive modelling in terms of mortality trends.”
Organizations in the U.K. were the first to use postal codes as a longevity indicator, with the evidence of correlation quite strong, says Monteiro. However, there’s less evidence the same correlation exists in Canada. “Our analysis suggests it isn’t that strong, whereas some of our competitors have suggested, their analysis suggests, it is strong,” he says. “So the jury is still a little bit out on that.”
As well, data in smaller doses is less useful, so smaller sized plans will have to find larger data sets that still have a good chance of representing their specific membership’s reality, he says.
Read: How are retirement solutions affected by wealthier Canadians living longer?
“An individual pension plan might be too small to just look at their own data,” says Simmons. “It’s helpful if you have thousands or even hundreds of thousands of records . . . to look at. A smaller pens-ion plan really needs to be reaching out to a service provider of some sort who’s done the work, not only for their plan but also aggregated it with lots of other data as well.”
It’s also key to revisit the data to ensure it’s updated to reflect any changes in the employee or retiree population, he says. “It depends on how quickly the population is turning over. . . . We try to subdivide them into different groups: Is there a unionized group and is there an office worker group? And what we find is that the experience within those groups doesn’t change too quickly, but you may see the relative size of those groups change.”
A little privacy, please
When it comes to member data, privacy is an important consideration for any pension plan, says Rivard.
Monteiro agrees, noting there are certain barriers when looking for more data to add to the mix. Information on individual’s health statuses would be useful, but there’s also a perception that this would be crossing a privacy line, he adds.
Read: How analyzing big data can help with pension engagement
“Obviously, if we did have something like someone’s smoking status or their specific lifestyle characteristics, that would be more powerful,” says Richard Brown, a longevity risk consultant at Club Vita, a subsidiary of Eckler Ltd. “But the pension plans can only collect information that is applicable to their role.”
On the other hand, benefits providers have better access to health-related information about employee groups, so Brown suggests they co-ordinate with pension providers to harness those valuable insights. “It is a potential opportunity if those privacy restrictions aren’t an issue,” he says. “You could potentially use that insight to get a better sense of longevity.”
Widening the net
As the idea of using big data gains popularity, pension plans and their consultants are looking for more information that’s already available and potentially useful, says Brown. “The ones that have really emerged within the pension and group annuity markets . . . are things like . . . looking at someone’s earnings level before they retired because that could give the better picture of someone’s affluence,” he says.
But pre-retirement earnings are a trickier piece of data to come by since it may not be tracked by an employer, or a plan member may not have been employed by the plan sponsor in question at the time of retirement, says Brown.
It’s also important to remember scenarios where pension recipients are a former employee’s surviving spouse or other beneficiary, says Brown, so the recipient’s gender is factored into life expectancy, as well as the fact that a spouse’s life expectancy decreases after the death of a partner. Indeed, married people are expected to live longer than single people, he says. Finally, whether a plan member is disabled at the time of retirement is also a factor, since it usually indicates a lower life expectancy.
Organizations in the U.K. have uncovered other data that further solidifies the connection between affluence and longevity, says Tom Ground, managing director of defined benefit solutions at Aviva. “There are lots of signs of conscientiousness that you can tell from each life. In the U.K. you have to register to get your car certified for the road every year. And we can check whether someone does that in a punctual way or in a late way. If they do it two days before, or after the date it was due, that determines the level of conscientiousness they might have.”
Fewer pensioners living longer
Canadian plans may be taking an overly conservative approach to their liabilities, according to Club Vita. Its measurement tool, which tracks more than 500,000 pensioners from 40 Canadian plans, found that, between 2005 and 2014, more people died than were expected by the widely used Canadian pensioners’ mortality study.
The negotiating table
For plans looking to de-risk, it’s a major advantage to have the most clear and accurate data when negotiating a price with an annuity provider, says Ground.
Read: Annuity sales hit record highs in 2018, led by buyouts: report
However, his firm often finds pension plans still have some way to go in analyzing their own data, so it always performs its own assessment based on what the plan’s collected and what else is available. Often, that assessment works out in the pension plan’s favour, says Ground.
The more data the better, notes Simmons. “[In] the industry as a whole, we’ll give as good a quote as we can based on the data that’s available. But if there’s not a lot of data available, we’re probably putting in some more margins, because maybe the data points are pointing in different directions, and we’re not 100 per cent crisp on what or how these folks’ longevity is going to evolve in the future.”
A plan sponsor with clear and plentiful data they’re willing to share is likely to get a better price for an annuity, he says, because insurers will feel more comfortable in underwriting the group.
Martha Porado is an associate editor at Benefits Canada.